Project summary
Conveyor belt systems are the arteries of many production lines and are critical in securing the material flow between processing units. Disruption of operation due to unplanned stops when a critical component fails leads to unexpected costs and in the worst case a stop in production and delivery to customers. Conveyor belt systems are used in virtually all processes industries, but also other sectors where bulk material must be transported. In mining operations, these systems are present throughout the complete production chain.
The CoCoP project develops and validates a break-through innovative and flexible SaaS solution for condition monitoring and health prediction of conveyor belt systems. Contrary to available solutions on the market, a system level approach is taken to understand wear and tear of critical components and to predict when the systems will fail. Combining data from all around the conveyor belt system with the physical behavior and operational characteristics, component degradation will be predicted and timely actions for operation and maintenance will be prescribed.
The core of the targeted solution is the Predge digital platform for predictive decision support where a further developed prototype solution for predictive maintenance of conveyor belt systems will be integrated and validated in life operation. The prototype uses a digital twin of the conveyor belt system combining domain knowledge with machine learning and AI principles.
The CoCoP project consortium consists of LKAB and Predge AB, where LKAB provides access and expertise on the facility for validating solution, and Predge AB provide the digital platform and the prototype. The joint effort targets a market-ready solution that increases utilization and reduces unplanned stops.
Concept & Approach
The CoCoP solution builds on the conceptual idea of a digital twin that replicates the behavior of a conveyor belt system in terms of its mechanical dynamics. The digital twin will contain a physics-based model that is representing the forces and moments acting on all components in the CBS and thereby getting and understanding of the degradation of the components. Clearly, the degradation is highly dynamic and depends on the operation of the CBS
The component models are then calibrated to the real-life system using data driven approaches. The condition monitoring of the real-life system is them performed by feeding all available data to the digital twin which is then assessing each component in the system by operating the twin online and quantifying the degradation rates and remaining useful life of the components.
In addition, CBS life can be compromised by the unwanted object entering the conveying system and need to be identified and alarmed for. A vision-based solution using an object classifying AI which is continuously monitoring the CBS.
Implementation
The project is organized into seven work packages as follows:
- WP01: Go-to-market strategy (M1-M12)
- WP02: Project management (M1-M12)
- WP03: Dissemination and communication (M1-M12)
- WP04: Test protocols, validation plan and operation campaigns (M1-M2)
- WP05: Conveyor belt system predictive decision support (M2-M8)
- WP06: Belt life hazard mitigation (M4-M9)
- WP07: Integration and validation (M4-M12)
The development work packages will engage after the initial planning and analysis in WP04 where the individual test campaigns and validation operation are laid out. WP05, WP06, and WP07 will perform the PDCA cycle managed by WP02. The logical flow is depicted in the following diagram
Three project KPIs are defined that are used to assess the performance of the solution relating in part to business benefits generated by the CoCoP solution:
- Project-KPI1: The predictive decision support solution for conveyor belt condition monitoring and health prediction is running in life operation for at least 3 months.
- Project-KPI2: Failures of critical components can be successfully replicated by the scenario-based assessment tool, with a success rate of 80%.
- Project-KPI3: The availability/utilization of conveyor belts is increased, and the aggregated downtime is reduced by 20% comparing two periods of operation with similar operational characteristics.
Project facts
Project run time: 1st August 2020 – 31st July 2021
Project cost: 294.000 €
Funding body: EIT RawMaterials
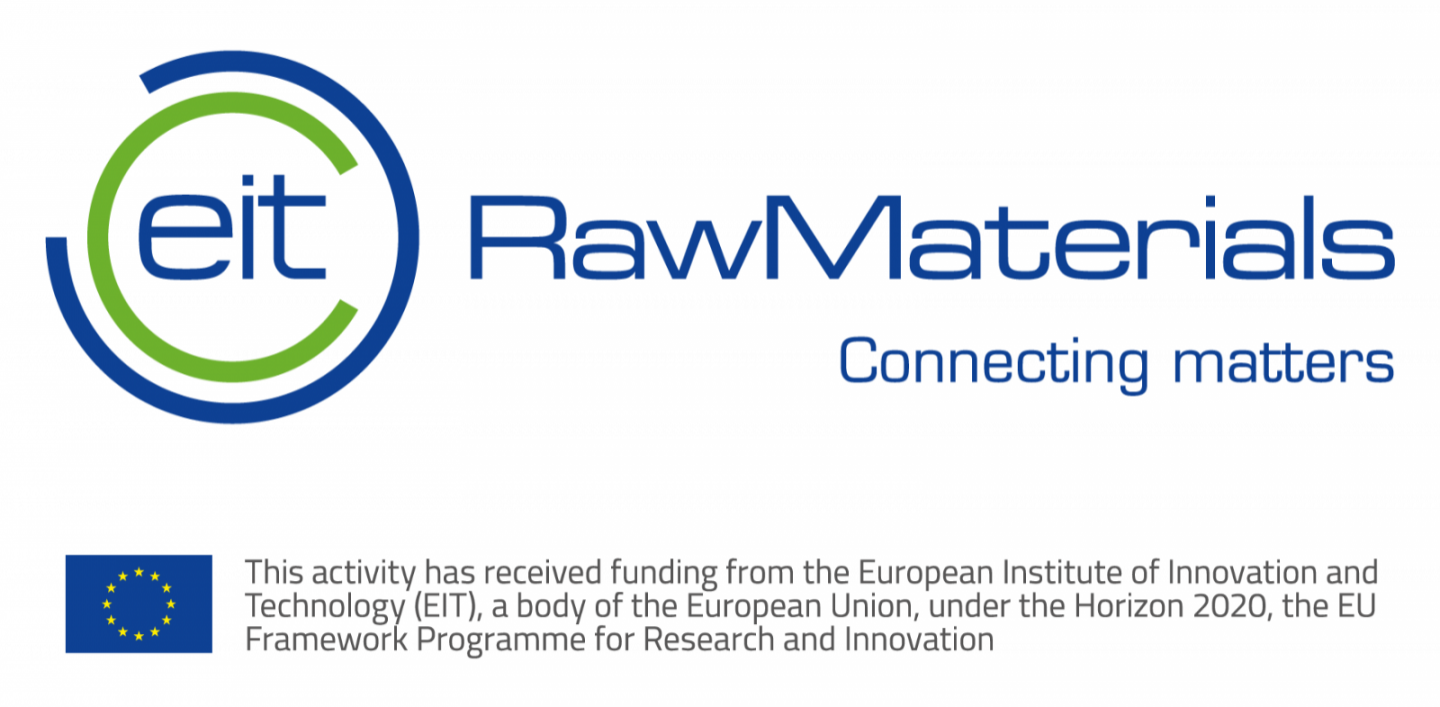